Building Legal AI Benchmarks That Matter: From Theory to Implementation
- Colin Levy
- 6 days ago
- 7 min read
AI tools in legal tech continue to advance rapidly, but as I've explored in my recent series on legal benchmarking, the gap between controlled demonstrations and real-world performance remains significant. LegalBench and similar public benchmarks offer valuable insights into AI's general legal reasoning capabilities, but they don't measure how these tools perform against your firm's specific workflows, document types, and standards.
This piece brings together key insights from my previous articles while focusing on the question I hear most frequently: "Yeah, but how do we actually implement benchmarks in our firm?"
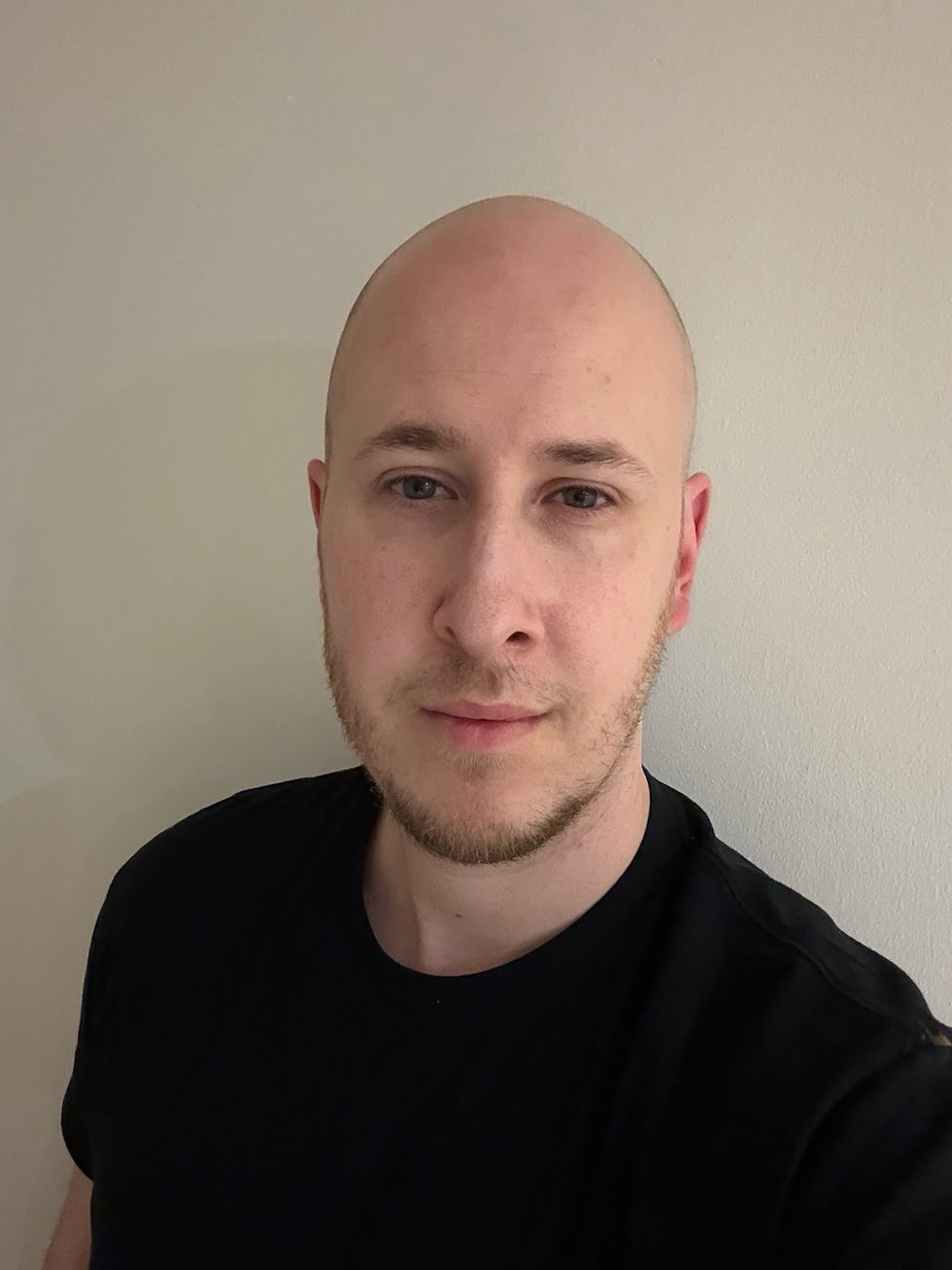
Why Standard Legal Benchmarks Aren't Sufficient
Public benchmarks like LegalBench test whether AI can handle legal reasoning tasks like statutory interpretation, case law analysis, and contract review. While valuable for understanding general capabilities, they don't address firm-specific challenges:
Your documents aren't the benchmark's documents.
AI might perform well on standardised contracts but struggle with your firm's bespoke agreements or industry-specific language.
Your workflows aren't the benchmark's workflows.
A tool that excels at extracting indemnity clauses might underperform at the cross-document reasoning your regulatory practice requires.
Your standards aren't the benchmark's standards.
What constitutes "good enough" varies dramatically by practice area, matter type, and risk profile.
Building internal benchmarks isn't just good practice: it's risk management. AI tools deployed without proper testing against your firm's actual work create potential liability that no general benchmark can mitigate.
The Overlooked Challenges Your Benchmark Should Test
Most benchmarks focus on simple accuracy: can AI extract this clause or identify that legal issue? But legal work involves more complex challenges that current AI often struggles with:
Multi-document reasoning:
Can AI connect related provisions across agreements, annexes, and schedules?
Imperfect inputs:
Does it handle scanned documents, handwritten annotations, and redacted sections?
Retrieval reliability:
When using RAG systems, does AI find the right legal content or just superficially similar text?
Document evolution:
Can it track meaningful changes across contract drafts?
Explainability:
Does it show its work, or just provide answers without supporting rationale?
These challenges represent the actual work of legal practice, not edge cases. If your benchmark doesn't test them, it's not testing reality.
The Three Phases of Benchmark Implementation
Building effective legal AI benchmarks isn't a monolithic project. It's a capability that develops through three distinct phases:
Phase 1: Lightweight Validation
Start small and focused. Please do not try to benchmark everything, identify one use case where AI could meaningfully impact your work.
What to build:
● 10-15 representative documents from your actual matters
● 3-5 specific questions the AI should answer
● Clear success criteria (e.g., "correctly identifies governing law clauses with 90% accuracy")
Who to involve:
● One legal subject matter expert who regularly handles these documents
● One technology-curious lawyer to oversee the testing
● IT support only as needed for secure tool access
When evaluating AI claims about functionality like "extract all key contract provisions," testing on your actual documents provides a more accurate assessment than using sample documents. Practice-specific documents often contain nuances that generalized tools aren't designed to handle.
Phase 2: Structured Evaluation
Once you've validated basic capabilities, expand to a more comprehensive benchmark covering a specific practice area.
What to build:
● 50-100 documents reflecting various complexity levels and formats
● Standardised evaluation templates for consistent assessment
● Test cases for the blind spots mentioned above (multi-document reasoning, imperfect inputs, etc.)
Who to involve:
● A practice group leader to establish evaluation standards
● 2-3 associates who regularly work with these documents
● Knowledge management or innovation staff to coordinate testing
At this stage, document both strengths and limitations. One firm testing a contract review tool found it performed well on standard agreements but struggled with their client's bespoke contract structures. This insight allowed them to implement the tool selectively, deploying it only where it demonstrated reliability.
Phase 3: Continuous Benchmarking
Transform your benchmark from a one-time evaluation into an ongoing capability.
What to build:
● A "living benchmark" that expands with new document types and edge cases
● Integration with your existing knowledge management processes
● Comparative benchmarks across multiple solutions
Who to involve:
● A dedicated legal AI evaluation function (can be part-time roles)
● Practice innovation leads from multiple groups
● Client-facing partners to ensure benchmarks reflect client needs
Continuous assessment allows firms to track how AI tools perform as they evolve and as your document set expands. As your benchmark matures, it becomes an increasingly valuable asset for guiding technology decisions.
Five Practical Steps to Start Next Week
If you're convinced benchmarking matters but aren't sure where to begin, here are concrete first steps:
1. Start with one high-value task
Don't try to benchmark "contract review" broadly. Focus on something specific like "identifying change of control provisions in credit agreements." Narrower scope means faster implementation and clearer results.
2. Use real documents, not hypotheticals
The most common benchmarking mistake is testing on clean, idealised documents rather than the messy reality of legal work. Use actual client documents (properly anonymised) that reflect your typical workflows, have a look in that abandoned filing cabinet in the corner of the office...
3. Define what "good enough" means before testing
Before running a single test, document what performance level would justify adoption. Is 80% accuracy sufficient if it saves significant time? Would you need 95% accuracy for high-risk documents? Setting these thresholds upfront prevents moving goalposts later.
4. Test what actually matters to your practice
Generic legal benchmarks test generic legal tasks. If your M&A practice has specific due diligence approaches, your benchmark should reflect those requirements. The question isn't whether AI is "good at legal tasks" but whether it's good at your legal tasks.
5. Document both capabilities and limitations
Don't just record what AI tools can do, explicitly document what they can't do reliably. These limitations often determine whether a tool can be safely integrated into workflows and also allow you to revisit them in the future after updates.
A Practical Example: Benchmarking Commercial Lease Analysis
Let's say your firm operates in multiple jurisdictions and regularly reviews commercial leases. Each jurisdiction has different statutory frameworks, terminology, and obligations. Your AI benchmark should test how well the tool:
● Extracts key clauses (rent review, break clauses, dispute resolution)
● Handles jurisdictional differences ("full repairing and insuring lease" in England vs. US lease structures)
● Integrates visual data (linking lease clauses to site plans)
Define scope and objectives:
● Break Clauses: Under what conditions can the lease be terminated?
● Rent Review Mechanisms: Is rent adjusted via CPI, fixed increases, or market review?
● Maintenance Responsibilities: Who maintains the property and common areas?
● Jurisdiction-Specific Requirements: How well does AI handle differences between UK and US leases?
Test multi-document reasoning:
If a break clause references another section, does the AI retrieve and interpret it correctly?
Test visual data alignment:
If a lease states "Tenant is responsible for maintaining Area A," does the AI correctly link it to the appropriate section of the site plan and flag it if Area A doesn't exist?
Test retrieval capability:
If the tool uses RAG, verify whether it retrieves the correct lease sections and relevant statutory provisions.
Hopefully this example show how we can move from abstract benchmarking concepts to concrete, practice-specific testing.
Common Implementation Pitfalls
Three common mistakes undermine otherwise well-designed benchmarking programs:
The Perfect Benchmark Fallacy
Some firms never implement benchmarks because they're designing the perfect, comprehensive evaluation framework. Don't let perfect be the enemy of started. A basic benchmark covering 20% of your needs but implemented next week delivers more value than a comprehensive framework that remains theoretical.
The Misaligned Benchmark
Benchmarks designed without proper practice input may test capabilities that don't align with actual workflows. Solutions may perform well on the benchmark but poorly in practice. Ensure your benchmark connects directly to daily work by involving practitioners who would use these tools.
The Static Benchmark
Legal practice evolves, and AI capabilities change rapidly. A benchmark that isn't regularly updated loses relevance quickly. Build in processes to refresh your benchmark with new documents, test cases, and evaluation criteria.
Making Benchmarks Matter: Organisational Integration
Technical benchmarking is only half the equation. For benchmarks to drive better decision-making, they need organisational integration:
Link to procurement processes
Use benchmark performance data to inform technology purchasing decisions. Benchmarks provide objective criteria when evaluating different solutions, helping you select tools that actually meet your requirements rather than those that simply demo well.
Connect to risk management
Benchmarks should inform appropriate guardrails. If your testing shows an AI tool struggles with multi-document reasoning, your implementation should include human review for those scenarios.
Inform training and adoption
Benchmark results highlight where lawyers need to maintain oversight and where they can trust AI assistance. This clarity dramatically improves adoption by setting realistic expectations.
From Skepticism to Strategic Adoption
The legal industry's initial AI skepticism is giving way to strategic adoption. Benchmarks provide the bridge between these positions, allowing firms to move beyond both blanket rejection and uncritical acceptance.
A rigorous benchmarking program provides the evidence needed to adopt AI with confidence, knowing exactly where it can deliver value and where human expertise remains essential. That clarity is worth the investment.
After all, the goal isn't implementing AI. It's delivering better legal services. Benchmarks ensure technology serves that purpose rather than becoming an expensive distraction.
As Head of Development in KPMG Law's - Global Legal Solutions team, Ryan McDonough specialises in bridging the gap between technology and law, focusing on crafting cutting-edge legal technology solutions. His expertise in creating efficient, user-friendly tools empowers clients with reliable legal resources. Driven by a passion for generative AI, Ryan leads pioneering initiatives that enhance legal frameworks to keep pace with rapid technological advancements.
With a rich background that includes roles such as Senior Software Engineer Team Lead at Addleshaw Goddard and Lead Web Developer at Epiphany Search, Ryan brings a wealth of experience in software development, DevOps, and team leadership. His contributions have streamlined development processes, led the introduction of automated systems, and spearheaded the development of large-scale web applications and AI-driven platforms. Ryan's work is characterised by a relentless pursuit of excellence and innovation in the legal tech sector.